Google Ads Recommendations
For what it’s worth: the views expressed below are shared by most top performing Google agencies. We are not alone.
The short version? Experienced professionals usualy IGNORE the Recommendations section in the Google Ads account. Yes, it results in a lower apparent account score, but it actually works better for our clients.
The Recommendations feature in the Google Ads platform is powered by Big Data collected from advertisers all over the world, combined with AI systems and decisioning processes. It’s designed to give some direction to the average Google Ads user as to what they “could do” to make the platform work more successfully for them. They are not a “must do”, but Google do try to make it compelling by scoring the campaign’s alignment with the recommendation. Anything below 100% means there is some Google Recommendation that is not being followed. This article is about why following the Recommendation is not necessarily going to make it work better.
When Recommendations are issued, you can either:
- Execute the recommendation as suggested
- Modify the recommendation and apply what you want from it
- Dismiss it entirely (but if you do, it will come back again after a few weeks to nag you)
Seeing a score against a campaign of just 50% could be worrying. Can you really make it perform twice as well? What if you’re an agency and your clients have access to the platform and see a 50% score on their campaigns; will they claim you don’t know what you’re doing if Google says you could do better? Will you get fired for your apparent incompetence for not getting it to 100%?
This does happen BTW.
Side note: Google Partner Agencies are incentivised with rewards and benefits to maintain a high platform score in the accounts they manage. RankPower is a Google Partner Agency. Read to the end of this article to find out our approach to this.
Here’s Our Take on the Function:
Remember doing Statistics when you were at school? You might remember learning about the Bell Curve.
A Bell Curve is a representation of data sets and how individual points is distributed within a typical set. In the case of my example below, I’m going to use it to represent the performance of a Google Ads account.
This is not an actual whitepaper, it’s just how I approach what I hope is a half-decent explanation of how and why Google Ads Recommendations are the way they are, and why most experienced operators think they are rubbish.
Back to the Bell Curve:
The space under the curve in my illustrations represent the likelihood that an account is performing at a given level. Loosely, I’m saying that there are some poor performing accounts (the low space before the hump), a whole bunch of average performing accounts (the middle section of the hump), and some high performing accounts (the low space after the hump).
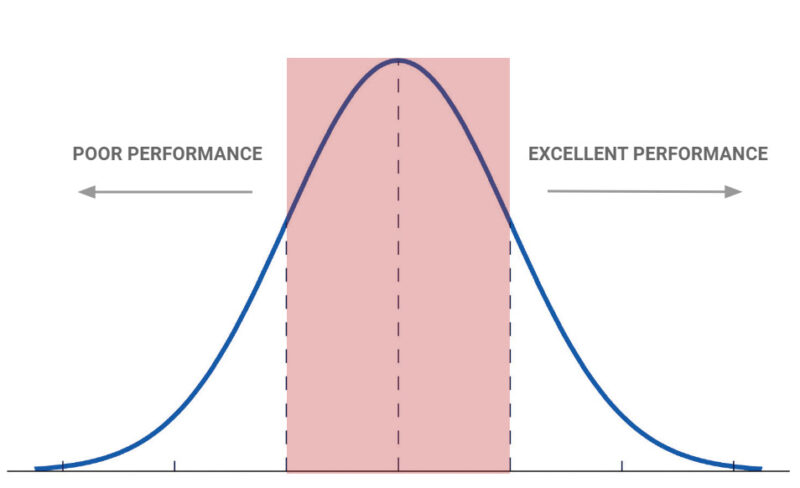
This is logical, because some advertisers are consistently winning more business from their search ads than others – they are the ones that outperform the average, dominate search in high rank positions, and probably have their businesses in growth mode. While others feel they are getting little from running ads at all.
The red section in Figure 1 represents the majority of accounts. It’s also the area where Google can gather the most data to support their AI engine. All accounts in similar verticals all around the world are adding to their massive dataset. This in turn helps identify what most accounts could be doing better, and what it might take to subtly shift that performance upward. It’s what many businesses in this section might benefit from.
Figure 2 below represents what I believe Google’s objective is. If they can subtly move the middle of that Bell Curve towards performing better, then not only will those businesses with average performing accounts directly benefit, but they will also come to feel more confident in the value delivered by Google Ads.
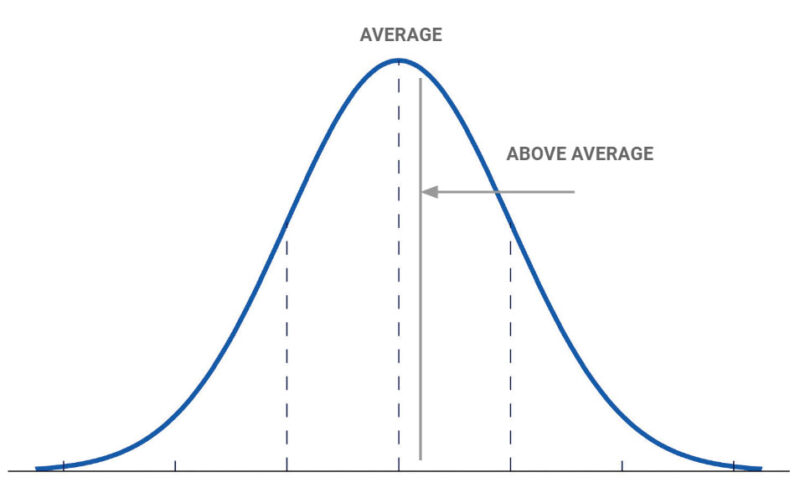
That last point is really the key.
“…to feel more confident in the value delivered by Google Ads”
This is what prompts businesses to invest more money.
I know it seems like a cynical view, but it really shouldn’t. The primary objective of any business is to make money, and if Google can see the opportunity to make more, why wouldn’t it take it up?
“More than 80% of Alphabet’s revenue comes from Google ads, which generated $147 billion in revenue last year. Google has been the market leader in online advertising for well over a decade and is expected to command nearly a 29% share of digital ad spending globally in 2021”
CNBC 2021-05-18 How Google’s $150 billion advertising business works
So, here’s my view on what could be achieved – just to get some of the Bell Curve performing just that little bit better to push the average up a couple of points into the “above average” zone.
Could this encourage many advertisers to invest more? I think yes. Is a performance shift even as small as 5% going to have the desired effect? Again, I think yes. Don’t forget, this is off Big Data, Masses of Advertisers, and Billions of Dollars are involved.
But who specifically is this going to help?
I was going to list some explicit examples of what type of operator fell into the “below average” category but changed my mind – let’s just say they are clearly “below average”. I will say however that even some reputable agencies fit into it, as high staff turnover or low salaries sometimes means a client ends up being serviced by a relatively inexperienced operator. Sadly, there is a very wide spectrum of relative “expertise” in this industry.
When it comes to following Recommendations, some operators (many of them?) will ignore them entirely. They can. There’s no rule that says they need to follow them. It means that some advertisers may benefit from the Recommendation, while others do nothing and stay where they are on the curve. Mixed levels of uptake create a subtle shift upwards of the average, not a huge bound forward. And as a result, some advertisers get better results and feel more confident in investing in Google Ads. Ergo: Google maybe makes a few billion more.
OK, so in Figure 3, this is the general effect: Some of the poor performers may lift their performance to average, or even a little above. Well done!
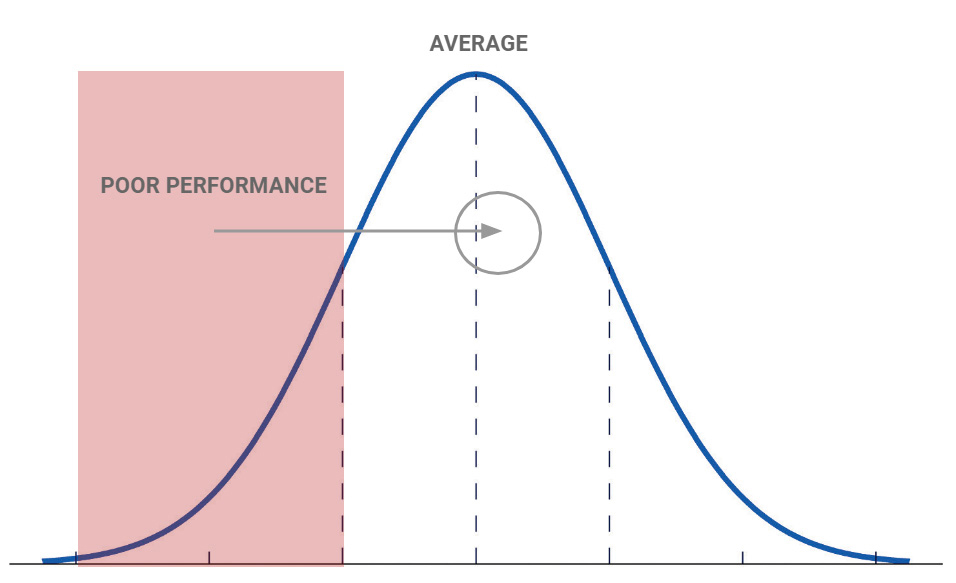
The Problem with the Google Ads Recommendations:
The key problem here is not how the Recommendations affect poor performing campaigns, we’ve seen that this is likely to be generally positive.
Recommendations are designed for the average or below average performing campaign. A lot of data goes into designing them. The AI has applied huge performance datasets to figure out what works. And yes, Recommendations are tailored to each advertiser’s account, but (and this is HUGE): The AI system doesn’t properly account for high performance.
Google’s systems can’t really identify clearly what accounts are exceptional performers because they are outliers on the Bell Curve. They are the accounts operating on the far-right-hand side of the Curve where the number of advertisers is far fewer than in the centre of the Curve.
This is a problem because:
Recommendations issued by Google will continue to assume an advertiser is “average” and try to get the performance to “above average”. But what if it’s “well above average” or even “exceptional”?
In such cases, it’s not unusual for the Google Ads Recommendation score to be at 70%, not 100%, despite the performance level – because generally Google doesn’t know enough about the advertised business to be able to figure it out. This could prompt some operators to apply a recommendation that degrades the account performance toward “above average”. Remember, they were operating in advance of that.
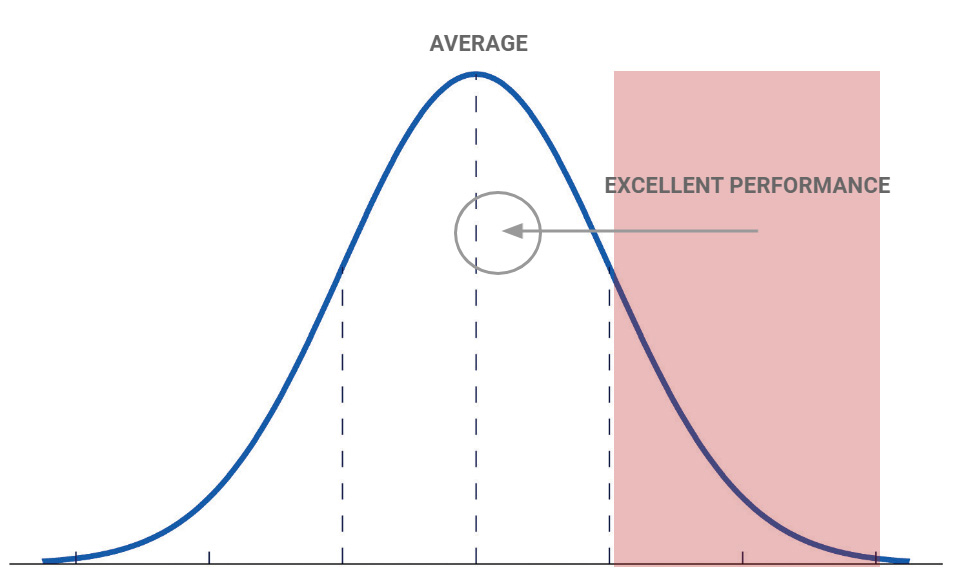
Also remember: Google incentivises Google Partner Agencies to apply recommendations. They can also choose to Dismiss them, but the nagging messages will just come back.
Worse still, if the client has access to the account, they may consider that Google is smarter than their agency and insist on applying the Recommendations. This is not uncommon. In fact, it happens often. A client may cause the adoption of a Recommendation that reduces an exceptional account downward toward above average, or worse. Agencies like RankPower are under pressure to justify why we think 70% (or even 50%) is better than 100%.
We do indeed check and dismiss them from time to time, but not for the sake of the score, rather as a reminder to ourselves to ‘consider and decline’ based on our experience and insight.
A frightening aspect of Google Ads Recommendations is that Google transitioned them to “auto apply” some time ago. So suddenly, exceptional accounts were being auto edited by Google to become just average.
Thankfully, Google now offers an option to auto-accept or deny any Recommendation, but not until after the performance of many optimal Ads account had been negatively affected.
So, what do we do at RankPower?
- We disallow almost all auto-apply Recommendations.
- We audit all other Recommendations and accept only those that make sense and will improve performance, based on our experience with our specific client’s needs.
- We ignore our Google Partner incentives and dismiss all other Recommendations that we don’t agree with.
- Sometimes Google scores us 70% and we don’t care.
Want to know more about this topic? Feel free to start a conversation.
This article is strictly copyright.